It’s remarkable how quickly generative AI has been integrated into the healthcare industry.
Just two years back, this would have been almost impossible to achieve. But then came ChatGPT’s meteoric rise, with 100 million users in 60 days; a moment that led numerous businesses to adopt AI into their business frameworks.
For the healthcare industry, the use cases of adopting Generative AI are too good to ignore. 24×7 customer service, data analysis, and the creation of synthetic patient data are some of the primary applications of generative AI within the industry.
For businesses, Generative AI unlocks an unparalleled potential to boost efficiency through its application. However, the healthcare industry has always been one of the slowest to adopt new technologies.
This often results in lower rates of implementation and a lack of skilled personnel specializing in the implementation of healthcare technology. The question is clear – how to build a generative AI solution for healthcare businesses?
The easiest option would be to embrace existing commercial Generative AI models. Popular Generative AI examples include GPT-4, LlaMA 2, Claude 2, and Gemini; these models offer customization options for businesses to benefit from.
However, healthcare businesses can also opt to custom-build their LLMs provided they have their data and technical infrastructure.
In this article, we will explore the nuances of choosing the right generative AI models as well as a step-by-step process of building a generative AI solution for your healthcare business.
Table of Content
- What is Generative AI and How Does it Work?
- Generative AI Use Cases for Healthcare
- How to Build a Generative AI Solution: A 7-Step Process for Healthcare Businesses
- Build your Generative AI Solution with Kanerika
- FAQs
What is Generative AI and How Does it Work?
Generative AI is a type of artificial intelligence that focuses on creating new content. This could be in the form of unique content, whether it be images, text, audio, or other types of data.
Generative AI examples include applications in diverse fields such as banking, finance, and healthcare.
How Does Gen AI Work?
Different models of Gen AI have their unique approaches. But they share a few common stages.
Here’s how Gen AI largely works:
Training
Generative AI models are trained on massive amounts of data, like text, images, or code. This data helps them learn the underlying patterns and relationships within that specific domain. Imagine an AI being shown millions of photographs of dogs.
Understanding
Through this training, the AI develops a deep understanding of the data’s characteristics. Such as the typical composition of a dog’s face, its fur patterns, or its common poses.
Creation
Once trained, the AI can then use this knowledge to generate entirely new content. It can create a photorealistic image of a dog wearing a hat, or write a poem about a dog’s adventures.
Read More – Everything You Need to Know About Building a GPT Models
Generative AI Use Cases for Healthcare
Generative AI, with its ability to create and analyze complex data, offers a multitude of applications in the healthcare sector. Here are some key use cases:
- 24×7 Customer Service: Implementing AI for customer service can significantly enhance patient engagement and support. It can provide round-the-clock assistance, answer queries, schedule appointments, and offer personalized health tips, thereby improving the overall patient experience.
- Creation of Synthetic Patient Data: To maintain patient confidentiality while enabling extensive research and development, generative AI can create synthetic patient data. This data mirrors real patient information without compromising individual privacy, offering a rich resource for medical research and training.
- Drug Discovery and Medical Research: AI accelerates the process of drug discovery by simulating and analyzing the effects of various compounds. This can significantly reduce the time and cost of developing new medications and therapies.
- Predictive Analytics for Preventative Care: By analyzing trends and patterns in health data, AI can predict potential health issues before they become serious, enabling proactive rather than reactive healthcare.
- Enhancing Diagnostic Accuracy with AI-assisted Imaging Analysis: AI algorithms can interpret medical images, such as X-rays, MRIs, and CT scans, with high precision, often detecting anomalies that may be overlooked in manual examinations.
Read More – Generative AI in Telecom: Use Cases and Benefits
How to Build a Generative AI Solution: A 7-Step Process for Healthcare Businesses
Generative Artificial Intelligence is revolutionizing healthcare, enhancing diagnostic accuracy and treatment efficacy.
As a result, its demand in the healthcare sector is on the rise! Valued at $2.4 billion in 2019, the Gen AI healthcare market is expected to touch $30 billion by 2025.
The reasons for its success are clear. AI algorithms have multiple use cases that can be very useful for healthcare businesses. For example, AI can interpret medical images to detect anomalies that may be missed by the human eye.
AI can also gradually assist doctors in diagnosing various illnesses at early stages. This can lead to more efficient medical procedures and healthier patients.
Here’s a step-by-step procedure of how to build a generative AI solution for the healthcare industry.
Step 1: Define Generative AI Goals for your Healthcare System
To effectively implement a Generative AI solution in healthcare, it is crucial to first identify the specific tasks it will undertake.
Prioritize functions that are either highly challenging or require significant human resources.
For each selected task, the AI must be trained on historical data to ensure accuracy and relevance. These are a few possible applications with the current state of Gen AI:
- Generative AI can meticulously analyze extensive medical data, and help in precise diagnosis of diseases. This capability is particularly valuable in identifying subtle or complex conditions.
- Utilizing AI technology, healthcare providers can develop personalized treatment plans. These plans are based on the unique medical history of each patient.
- By integrating AI into hospital operations, there is a significant opportunity to streamline administrative processes.
- In the realm of drug discovery and medical research, AI has the potential to significantly expedite the development process.
Selecting the right tasks for your Generative AI solution involves focusing on areas that present the greatest challenges.
Step 2: Understand your Healthcare Data Collection and Data Infrastructure
Begin the process of building a generative AI solution by gathering relevant data that will fuel the algorithm. The efficacy of these AI models hinges on the quality of the data they are trained on.
High-quality data, free from errors and inconsistencies, is essential for the accuracy and reliability of AI-driven decisions. Should the data contain inaccuracies or biases, the resulting outputs could be unreliable, potentially leading to erroneous treatments.
This is especially vital for the healthcare industry where any discrepancies in data can lead to negative outcomes for patients.
Strict Data privacy laws have also led to most healthcare institutions using synthetic data for electronic health records. This ensures that replicas of electronic health records (EHRs) of patient data are used to ensure patient confidentiality. Proper data storage is equally important to prevent any leaks from the healthcare systems.
Moreover, strict adherence to regulatory standards, such as HIPAA and GDPR, is crucial in safeguarding sensitive health information. These regulations ensure that patient data is handled with the utmost confidentiality and security, maintaining trust and integrity in healthcare AI applications.
Step 3: Choose the Right AI Platforms and Tools
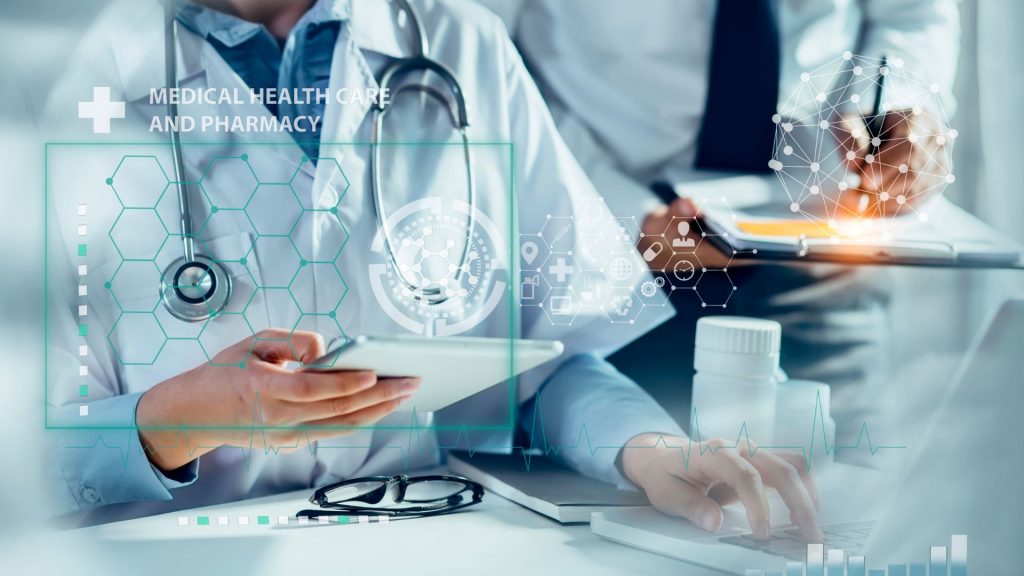
There is now an abundance of AI-led tools in the healthcare industry
Not all Gen AI platforms are created equal. For example, some platforms excel at natural language processing, making them ideal for chatbots and virtual assistants. Others shine in data analysis, aiding in clinical decision-making and risk prediction.
For healthcare applications, you may want to consider the following generative AI solution providers:
IBM Watson Health (Formerly Merative L.P.)
Known for its powerful AI capabilities, IBM Watson Health offers solutions for data analysis, clinical decision support, and operational efficiencies. It’s particularly strong in processing natural language and can integrate with various healthcare systems.
Vertex AI from Google
This platform provides advanced AI tools tailored for healthcare. It excels in data management, and analysis, and offers solutions for imaging, diagnostics, and predictive analytics. You can build an AI system using Vertex and leverage Google’s Healthcare Data Engine and context-aware search for quick deployment.
Microsoft Azure Health Bot
Azure offers AI services that can be used to create interactive healthcare bots. These bots can assist in patient engagement, and triage, and provide personalized medical information. Additionally, the AI-based health insight tool offers three different models. These models assist clinicians in reviewing patient histories, simplify medical reports for patient comprehension, and identify discrepancies in radiology reports.
Nuance Dragon Ambient eXperience (DAX)
DAX utilizes Ambient Clinical Intelligence to securely capture and contextualize physician-patient conversations during visits. This technology operates in the background, allowing clinicians to focus on the patient rather than on taking notes. By reducing the time clinicians spend on documentation, DAX allows for more direct and meaningful interaction with patients.
Popular Generative AI Solutions
Besides TensorFlow and PyTorch; OpenAI, Google Gemini, and LLaMA offer different types of generative models, libraries, and machine learning platforms. They are highly customizable and choosing the right one is a fundamental step in how to build a generative AI solution successfully.
Note that you cannot build a custom AI for your organization if you choose any of these tools but you can certainly train available models on data that make it more relatable for your organization.
Step 4: Hire Consultants or Get the Right Talent to Build a Healthcare-Focused Generative AI Solution
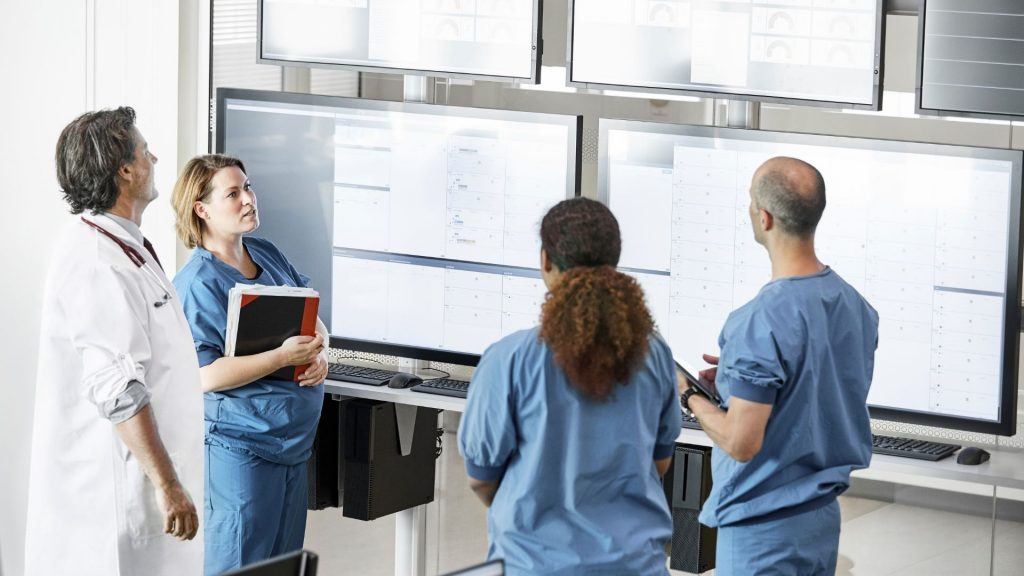
Healthcare businesses need the right team to make the most of their generative AI implementation
For a successful Generative AI project in healthcare, assembling the right team is crucial. This involves hiring skilled professionals and consultants who specialize in building a generative AI solution.
Before hiring keep these aspects in mind:
- Determine the specific skills needed for your project. This may include AI and machine learning expertise, data science, software development, and knowledge of healthcare systems.
- Seek professionals who have experience in healthcare AI. This includes understanding medical data, regulatory compliance (like HIPAA), and patient privacy concerns.
- Collaborating with universities or research institutions can provide access to cutting-edge medical research.
- Consider investing in training for your existing staff. Upskilling your healthcare IT team in AI and data analytics can be a cost-effective way to build internal expertise.
Step 5: Create an Effective Proof of Concept (PoC) for Your Generative AI Solution
Before you build a generative AI solution and train it, you need a prototype. Here is how to build a generative AI application PoC.
- Choose a specific, manageable use case for the PoC. This should be a representative scenario where the AI solution can have a significant impact. For example, a PoC might focus on using generative AI applications to analyze radiology images to detect bone fractures.
- Collect and prepare the data required for the PoC. This could involve anonymized imaging data. Ensure the data is of high quality and representative of the real-world conditions the AI will encounter.
- Configure the AI model to address the chosen use case. This involves training a machine learning model on the collected data. An expert would have to guide it through the first several thousand images.
- Run the PoC in a controlled environment to evaluate its performance. Monitor how well the AI solution works in terms of accuracy.
It is time-consuming and can take many months but thereafter the system keeps fine-tuning itself. Think of the system as an infant that learns about the alphabet, reads a few books, and then starts writing.
Step 6: Evaluate and Check for Biases Within Your Healthcare AI Model
AI model bias is a significant challenge that can lead to inexact outcomes.
For example, if an AI system used in a hospital is trained mostly with medical data from male patients, it might not be as good at diagnosing diseases in female patients. It is not that men and women have entirely different physiology but older women have significantly more fractures than men due to osteoporosis.
To correct AI model bias take these steps:
- Ensure the training data is diverse and representative of the population the model will serve.
- Embed ethical considerations and fairness criteria into the AI development process.
- Regularly test the AI models for bias by checking the model’s outputs against various demographic groups
Building an AI algorithm must happen with transparency and explainability in mind. Understanding how and why a model makes certain decisions is crucial for identifying and correcting biases.
Bias correction is not a one-time task. Continuous monitoring and updating of the AI models are necessary.
Step 7: Maintain Your Generative AI Solution and Check for Improvements
The healthcare domain is evolving at light speed. With the very public success of ChatGPT, a new domain has opened up for investors and researchers alike.
Building an AI algorithm is no longer the rocket science it used to be a decade ago. New advancements are happening almost daily.
In this context, you need to regularly check for improvements to ensure its long-term effectiveness and relevance. We suggest a three-step approach.
- Regularly monitor the performance of your AI system. This includes tracking its accuracy, speed, and how well it’s meeting user needs.
- AI models can become outdated as new data becomes available or when user needs change. Periodically retrain your models with updated data to maintain their accuracy and relevance.
- Collect and analyze feedback from the users of your AI system, such as healthcare professionals and patients. Their insights can provide valuable information on building an AI algorithm that works efficiently.
Build your Generative AI Solution with Kanerika
Kanerika stands out as an ideal partner for creating generative AI solutions for businesses, offering a blend of innovation, expertise, and a proven track record.
Kanerika’s extensive expertise in AI/ML, combined with comprehensive data management and robust analysis capabilities, positions it uniquely in the US as a leading AI implementation partner.
Starting with a thorough pre-assessment of your needs, Kanerika’s team conducts an in-depth analysis to provide tailored recommendations for your healthcare business. Kanerika’s strategic alignment ensures scalability and future-proofing of solutions, while its collaborative approach guarantees that the solutions align perfectly with your business objectives.
Choose Kanerika, and take a significant step towards building an AI algorithm that exactly matches your needs.