What happens when the algorithms we trust to make crucial decisions—like who gets a job or how medical resources are allocated—are flawed or biased? The concept of Responsible AI becomes vital in answering this question. According to a report by MIT Sloan and BCG, while 52% of companies claim to adopt responsible AI practices, a significant majority (79%) of these companies admit that their efforts are restricted to small-scale, pilot, or limited implementations, and have yet to achieve broader organizational impact.
As AI systems increasingly influence critical aspects of our lives, ensuring they operate responsibly is more than a technical challenge—it’s a moral necessity. In this blog, we’ll explore how Responsible AI can ensure fairness, transparency, and accountability in AI-driven decisions.
What is Responsible AI?
Responsible AI refers to the development and deployment of artificial intelligence systems in an ethical, transparent, and accountable manner. It encompasses principles and practices that ensure AI technologies benefit society while minimizing potential harm. Responsible AI focuses on fairness, privacy, security, and interpretability of AI systems.
For example, consider an AI-powered hiring system. A responsible approach would involve:
- Ensuring the training data is diverse and free from historical biases
- Making the decision-making process transparent and explainable
- Regularly auditing the system for fairness across different demographic groups
- Protecting applicant data privacy and security
- Maintaining human oversight in the hiring process
An irresponsible implementation might use biased historical data, leading to discriminatory hiring practices, or make decisions without any explanation, leaving applicants in the dark about why they were rejected.
Responsible AI aims to harness the power of AI while upholding ethical standards and societal values.

Why Are Ethical Standards Important in AI Development?
As AI becomes increasingly integrated into our daily lives, the concept of Responsible AI has never been more critical. It’s about striking a delicate balance between harnessing the transformative power of AI and ensuring that these technologies don’t inadvertently harm individuals or society at large.
From algorithmic bias that can perpetuate societal inequalities to privacy concerns surrounding data collection, the ethical implications of AI are vast and complex. As we stand at the crossroads of innovation and ethics, it’s crucial to understand how Responsible AI can shape a future where technological advancement goes hand in hand with human values and societal well-being. The following are the principles governing Responsible AI:
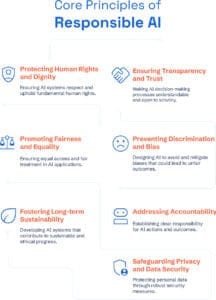
1. Protecting Human Rights and Dignity
AI systems can significantly impact people’s lives. Without ethical guidelines, these systems might infringe on basic human rights or dignity. Ethical considerations ensure AI respects and upholds these fundamental values.
2. Preventing Discrimination and Bias
AI systems learn from data, which can contain historical biases. Ethical development practices help identify and mitigate these biases. This prevents AI from perpetuating or amplifying discrimination in society.
3. Ensuring Transparency and Trust
Ethical AI practices promote transparency in how AI systems make decisions. This builds trust between AI developers, users, and the general public. Trust is essential for the widespread adoption and acceptance of AI technologies.
4. Safeguarding Privacy and Data Security
AI often requires vast amounts of data to function effectively. Ethical considerations in AI development ensure this data is collected, used, and stored responsibly. This protects individual privacy and prevents misuse of personal information.
5. Promoting Fairness and Equality
Ethical AI development strives to create systems that are fair and equitable for all users. This is crucial in applications like lending, hiring, or criminal justice, where AI decisions can have significant consequences on individuals’ lives.
6. Addressing Accountability
Ethical frameworks help establish clear lines of accountability in AI systems. This is important when determining responsibility for AI-made decisions, especially in cases where things go wrong.
7. Fostering Long-term Sustainability
By considering ethics in AI development, we ensure these technologies contribute positively to society in the long run. This sustainable approach prevents potential negative consequences that could hinder AI’s progress and acceptance.
Industry Initiatives and Standards
1. IEEE Global Initiative on Ethics of Autonomous and Intelligent Systems
The IEEE Global Initiative aims to ensure that every stakeholder involved in the design and development of autonomous and intelligent systems is educated, trained, and empowered to prioritize ethical considerations.
- Provides a comprehensive framework for ethically aligned design
- Offers guidelines across various domains including personal data, reframing autonomous weapons, and economic impacts
- Promotes transparency, accountability, and privacy in AI systems
- Regularly updates its guidelines to keep pace with technological advancements
2. EU Guidelines for Trustworthy AI
The European Union has developed guidelines for trustworthy AI to ensure that AI systems are legally, ethically, and technically robust while respecting fundamental rights.
- Emphasizes seven key requirements – human agency and oversight, technical robustness and safety, privacy and data governance, transparency, diversity and non-discrimination, societal and environmental well-being, and accountability
- Provides assessment lists for practical implementation
- Aims to foster public trust in AI technologies
- Serves as a basis for potential future AI regulations in the EU
3. OECD AI Principles
The Organization for Economic Co-operation and Development (OECD) has established AI principles to promote innovative and trustworthy AI that respects human rights and democratic values.
- Focuses on five complementary values-based principles for responsible stewardship of trustworthy AI
- Emphasizes inclusive growth, sustainable development, and well-being
- Provides recommendations for national policies and international cooperation
- Adopted by 42 countries, forming a global standard for AI development
4. Corporate AI Ethics Boards and Initiatives
Many leading tech companies have established AI ethics boards and initiatives to guide their AI development and ensure responsible practices.
- Google’s Advanced Technology External Advisory Council (dissolved, but principles remain)
- Microsoft’s AI ethics committee, AETHER (AI, Ethics, and Effects in Engineering and Research)
- IBM’s AI Ethics Board
- Salesforce’s Office of Ethical and Humane Use of Technology
These corporate initiatives typically:
- Develop company-specific AI ethics guidelines
- Review AI projects for potential ethical issues
- Provide recommendations for responsible AI development and deployment
- Engage with external experts and stakeholders on AI ethics issues

The Need for Responsible AI in Different Sectors
1. Responsible AI in BFSI (Banking, Financial Services, and Insurance)
In the BFSI sector, Responsible AI is important in ensuring fairness in lending, fraud prevention, and enhancing customer experiences. For example, AI systems for credit scoring or loan approvals should be designed to avoid biases that unfairly exclude certain demographics. Also, while striving for accuracy with privacy concerns in mind, AI-driven fraud detection systems must straddle the fine line between scrutiny and false positives.
2. Responsible AI in Manufacturing
AI-responsible manufacturing uses artificial intelligence to optimize production processes, improve quality control, and enhance worker safety without violating ethical standards. This involves ensuring that decisions made by AI systems are explainable and accountable, such as production schedule changes or employee safety matters. Furthermore, predictive maintenance through artificial intelligence also needs to be in line with sustainability objectives by reducing waste and energy consumption.
3. Responsible AI in Logistics
Logistics activities involve optimizing supply chains using Artificial Intelligence (AI), inventory management, and reducing delivery time frames. When it comes to resource allocation and workforce management issues, especially regarding transparency, all stakeholders should practice fair treatment. In addition to optimizing routes for emissions reduction purposes, ethical AI can reduce unfair labor practices such as overworking drivers based on biased algorithms, thus improving the environmental sustainability of logistics operations.
4. Responsible AI in Retail
Retailers employ personalization systems on their websites that utilize big data analytics tools like machine learning to create tailored marketing campaigns aimed at a particular group of customers based on their preferences. Responsible AI ensures that retail personalization algorithms do not inadvertently discriminate against certain customer groups and that customer data is handled carefully. Moreover, this includes misleading marketing strategies because an IA will suggest at least ethical recommendations.
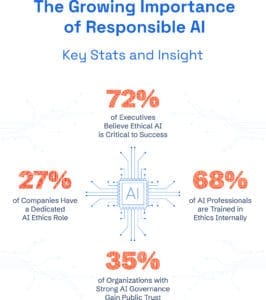
5. Responsible AI in Healthcare
Responsible artificial intelligence plays a significant role in the healthcare industry, where patients’ lives are at stake. Consequently, when it comes to diagnosing, treatment recommendations, and patient monitoring, AI systems should undergo thorough vetting to avoid biases that may cause misdiagnoses or unfair treatment. Furthermore, these systems must operate transparently for healthcare providers to be able to comprehend and explain AI-driven decisions to patients. In addition, ensuring privacy and security of patient data is a critical part of Responsible Artificial Intelligence.
6. Responsible AI in Pharma
AI is extensively used in the pharmaceutical sector, mainly for drug discovery, clinical trials, and personalized medicine. The ethical use of AI in this sector means that any form of bias resulting from such research has been eliminated, and clinical trial data are used ethically. Researchers and regulators must be transparent since this will enable them to understand why decisions were made based on artificial intelligence during drug development. This way, trust can be built, and good public health practices can be ensured through AI.
7. Responsible AI in Legal
The legal industry also utilizes artificial intelligence on some tasks like contract analysis or even predicting case outcomes. For example, responsible AI ensures that these systems are fair and do not perpetuate existing biases within the legal system. For instance, designing AI for sentencing recommendations to avoid re-entrenching discriminatory practices requires careful consideration. Also, lawyers and judges need insights they can trust; hence, transparency concerning such information is essential amidst all other things you shall receive from imperfect humans who have imperfect knowledge of your life circumstances with limited values they apply in making choices without empathy towards others except themselves.
8. Responsible AI in Other Fields
Other industries like education, public welfare, and energy also benefit from responsible AI. For instance, AI can offer personalized learning processes, but it must be done without bias or discrimination. Similarly, AI can help make decisions for public policy and resource allocation, but this has to be transparent and accountable so as not to enable the wrong use of power. Also, regarding the energy sector, AI could lead to more efficient resource utilization and lower emissions, although it must align with sustainability objectives so that it remains advantageous to the entire society as a whole.

Best Practices for Developing Responsible AI
1. Diverse and Inclusive Development Teams
Creating diverse and inclusive AI development teams is crucial for building responsible AI systems. These teams bring varied perspectives, experiences, and backgrounds, which help identify and mitigate potential biases in AI algorithms and applications.
- Include team members from different genders, ethnicities, and cultural backgrounds
- Incorporate individuals with diverse educational and professional experiences
- Ensure representation from various age groups and abilities
- Foster an inclusive environment that encourages open discussion and idea sharing
2. Rigorous Testing and Validation Processes
Implementing thorough testing and validation processes is essential to ensure AI systems perform as intended and don’t produce unintended consequences. This practice helps identify potential issues before deployment and maintains the system’s reliability.
- Conduct extensive testing across various scenarios and edge cases
- Utilize diverse datasets to validate AI performance across different demographics
- Implement adversarial testing to identify potential vulnerabilities
- Perform regular bias audits to detect and address any unfair outcomes
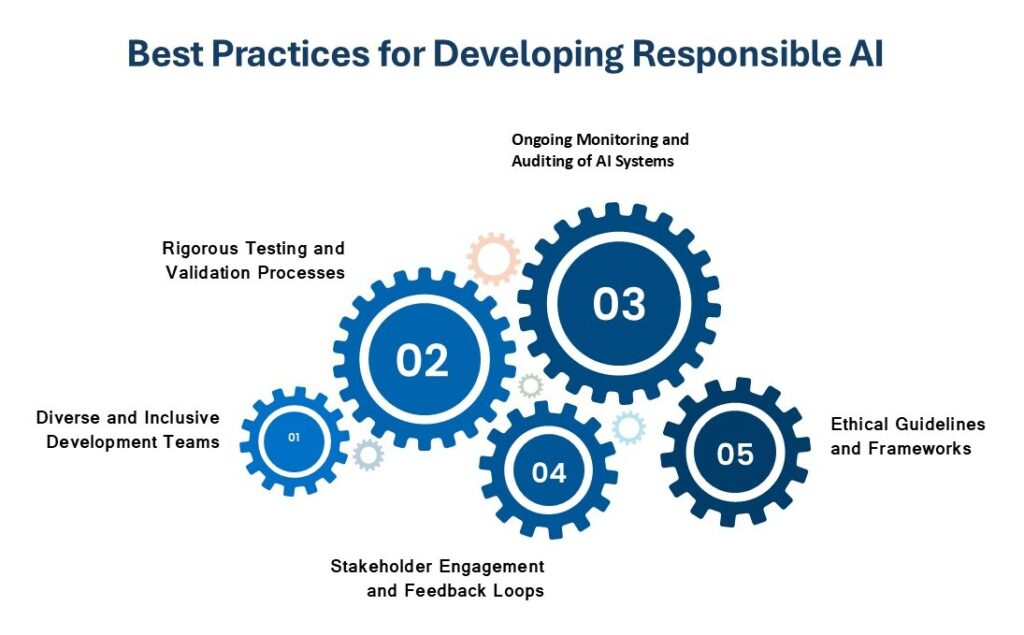
3. Ongoing Monitoring and Auditing of AI Systems
Continuous monitoring and auditing of AI systems after deployment is crucial for maintaining their responsible operation. This practice helps identify and address issues that may arise due to changes in data patterns or societal norms over time.
- Implement real-time monitoring systems to track AI performance and outputs
- Conduct regular audits to assess fairness, accuracy, and ethical compliance
- Establish clear processes for addressing identified issues or anomalies
- Maintain detailed logs of system behavior for transparency and accountability
4. Stakeholder Engagement and Feedback Loops
Engaging with stakeholders and establishing feedback loops is vital for ensuring AI systems meet the needs and expectations of all affected parties. This practice helps in identifying potential issues and improving the system based on real-world experiences.
- Identify and engage with all relevant stakeholders, including end-users and affected communities
- Establish clear channels for feedback and concerns
- Regularly solicit input on AI system performance and impact
- Incorporate stakeholder feedback into system improvements and updates
5. Ethical Guidelines and Frameworks
Developing and adhering to ethical guidelines and frameworks provides a solid foundation for responsible AI development. These guidelines help teams navigate complex ethical considerations and ensure consistency in decision-making.
- Establish clear ethical principles and values for AI development
- Create detailed guidelines for addressing common ethical dilemmas
- Implement decision-making frameworks for resolving ethical conflicts
- Regularly review and update guidelines to reflect evolving ethical standards and technological advancements

Tools and Resources for Implementing Responsible AI
1. AI Fairness Toolkits
AI fairness toolkits help developers identify and mitigate bias in AI systems. These tools are crucial for ensuring non-discriminatory AI applications.
- IBM’s AI Fairness 360: Open-source toolkit for detecting and mitigating bias in machine learning models
- Google’s What-If Tool: Allows visual inspection of machine learning model behavior
- Microsoft’s Fairlearn: Improves fairness and performance of AI systems
- Aequitas: Open-source bias audit toolkit for machine learning developers
These toolkits typically offer features like bias detection algorithms, fairness metrics, and mitigation techniques to help create more equitable AI systems.
2. Model Interpretability Frameworks
Model interpretability frameworks make AI decision-making processes more transparent and understandable to humans.
- LIME (Local Interpretable Model-agnostic Explanations): Explains predictions of any machine learning classifier
- SHAP (SHapley Additive exPlanations): Unifies various explanation methods
- InterpretML: Microsoft’s open-source package for training interpretable models and explaining blackbox systems
- Alibi: Open-source Python library for ML model inspection and interpretation
These frameworks provide tools for generating explanations, visualizing feature importance, and understanding model behavior across different scenarios.
3. Privacy-enhancing Technologies
Privacy-enhancing technologies help protect sensitive data while still allowing for effective AI development and deployment.
- TensorFlow Privacy: Library for training machine learning models with differential privacy
- PySyft: Open-source library for secure and private deep learning
- OpenMined: Community-driven project for privacy-preserving machine learning
- Microsoft SEAL: Homomorphic encryption library
These technologies enable secure data sharing, encrypted computation, and privacy-preserving machine learning, addressing key concerns in responsible AI development.
4. AI Ethics Guidelines and Checklists
AI ethics guidelines and checklists provide frameworks for considering ethical implications throughout the AI development lifecycle.
- IEEE’s Ethically Aligned Design: Comprehensive guidelines for ethical AI development
- EU’s Assessment List for Trustworthy AI: Practical checklist for implementing trustworthy AI
- Google’s Responsible AI Practices: Detailed recommendations for AI practitioners
- Microsoft’s Responsible AI Resources: Tools and guidelines for implementing responsible AI practices
These resources offer structured approaches to identifying and addressing ethical considerations in AI projects, helping teams integrate responsible practices into their development processes.

Case Studies: Top Companies’ Initiatives and Approach to Responsible AI
1. Google’s AI Principles in Action
Google was one of the first major companies to develop and publicly commit to AI principles in 2018. These principles aim to ensure that AI technologies are developed ethically, especially in terms of fairness, accountability, and transparency. To follow these principles, Google has a structured governance framework, including an AI ethics review board and integrating ethical considerations into product development processes.
For example, fairness checks have been implemented within products such as Google Translate to address issues related to gender bias, while tools like the What-If Tool enable developers to explore different factors’ implications on the outcomes of AI models. Additionally, Google continually updates the public on its progress, for instance, through annual publications like “AI Principles Progress Update,” which monitors this adherence across the company’s native intelligence projects.
2. Microsoft’s Approach to Responsible AI
Microsoft has also been at the forefront of advocating for Responsible AI. It has developed its own set of fairness, reliability, and privacy principles. The AETHER (AI and Ethics in Engineering and Research) Committee is key in ensuring that these principles are integrated into the development and deployment stages of artificial intelligence systems across the firm.
Some practical initiatives include embedding techniques that correct for bias within their AIs alongside explanation tools such as the Azure Machine Learning Interpretability Toolkit created by Microsoft.
Moreover, this company’s participation in international deliberations on ethical aspects related to artificial intelligence demonstrates how dedicated it is to establishing global standards governing the use of this technology.
3. IBM’s Commitment to Ethical AI
IBM has been a long-standing advocate for ethical Artificial Intelligence (AI), which would be trusted, transparent, and rooted in human values. IBM’s approach towards Artificial Intelligence (AI) ethics asserts ideals like transparency, explainability, and accountability. Among other things, IBM created tools such as AI Fairness 360 or AI Explainability 360 meant for detecting biases inside AI models.
Moreover, an emphasis has been placed on data privacy and security during AI development. Their research kit for AI Privacy and Security is a library that developers can use to ensure their machine learning systems are responsible for data. IBM’s ongoing research in AI ethics and collaboration with academic institutions and industry partners further strengthens its commitment to Responsible AI.
4. Facebook’s Approach to Responsible AI
Facebook has undertaken various initiatives towards responsible artificial intelligence, especially in content moderation and algorithmic transparency measures. The firm set up an AI ethics review board whose responsibility is to oversee the development and deployment of the systems, assuring they are aligned with ethical standards.
Meta’s fairness of algorithms projects focus on reducing biases to promote more bias-free models. This company’s creation of explainable AI tools also demonstrates how this giant technology player supports user understanding and regulatory comprehension in AI decision-making.
5. Salesforce and Ethical AI Practices
In developing its products, Salesforce has embraced the responsible use of artificial intelligence by integrating ethical considerations into CRM solutions. The Corporation has put forward its principles governing the ethical use of Artificial Intelligence throughout its operation dynamics.
Salesforce manufactures the Einstein AI tool, which has built-in mechanisms to identify and correct prejudices. This helps users create justifiable and fair AI solutions. The Corporation conducts regular reviews of its own artificial intelligence systems and ensures that they comply with ethical rules. The firm also collaborates with other organizations working towards Responsible AI globally.

Future Trends in Responsible AI
1. Advancements in Explainable AI
The need for Explainable AI (XAI) is rising as AI systems get more complex. The paramount concern of explainable AI is to make the decision-making process of a machine transparent and understandable to humans. Future developments in XAI will probably be about creating much-advanced tools and techniques that enable users to interpret and trust AI decisions. This will be particularly important in sectors such as healthcare or finance, where comprehending why an AI has made a certain decision is very crucial for accountability and trust.
2. Federated Learning and Privacy-preserving AI
Federated learning, an emerging method, allows AI models to be trained on decentralized devices or servers, which hold local data samples without sharing the raw data amongst them. This method, combined with other privacy-preserving techniques like differential privacy and homomorphic encryption, plays a crucial role in ensuring user privacy remains intact while AI systems function efficiently. As privacy concerns continue to rise, the importance of these methods cannot be overstated.
3. AI Ethics Education and Training Programs
The significance of ethics in artificial intelligence development would imply a considerable drive towards including ethics programs in academic institutions and training programs. This shift may well see dedicated curricula developed at universities and professional training courses focusing on ethical implications associated with artificial intelligence usage. These programs aim to equip future developers, data scientists, and policymakers with knowledge and tools that could lead to the establishment of fair, transparent, societally minded artificial intelligence systems.
4. Global Collaboration on AI Governance
The need for global cooperation in governing artificial intelligence will become increasingly critical as these technologies evolve and impact societies worldwide. Just as international treaties exist around climate change issues or human rights, there is a growing need for global frameworks and standards for AI ethics. Establishing such collaborative initiatives will involve governments, international organizations, academia, and the private sector working together to ensure that the development of artificial intelligence is characterized by shared ethical principles and addresses global challenges such as bias, transparency, and accountability.

Kanerika: Driving Enterprise Success with a Focus on Responsible AI
Kanerika is a leading AI company dedicated to driving business innovation and growth through the implementation of advanced AI solutions. We specialize in offering personalized AI strategies tailored to the unique needs of our clients across various industries, including BFSI, Manufacturing, Logistics, and Retail. Our cutting-edge AI solutions are designed to enhance efficiency, optimize processes, and unlock new opportunities for our clients.
At Kanerika, we prioritize data security and responsible AI implementation. Our commitment to these principles is reinforced by our ISO 27701 and 27001 certifications, which demonstrate our adherence to the highest standards of security and compliance. These certifications underscore our dedication to protecting our clients’ data while delivering AI solutions that are ethical, transparent, and aligned with global standards.
By focusing on Responsible AI, Kanerika ensures that our AI technologies not only drive success but also contribute positively to society. Our clients can trust us to deliver AI-driven innovations that are secure, compliant, and tailored to their business needs, helping them stay ahead in a rapidly evolving digital landscape.

Frequently Answered Questions
What is a responsible AI?
Responsible AI isn’t just about building smart systems; it’s about building *ethical* ones. It means designing and deploying AI that avoids bias, ensures fairness, protects privacy, and is transparent in its decision-making. Ultimately, it prioritizes human well-being and societal benefit over technological advancement alone. It’s about making sure AI serves humanity, not the other way around.
Which are the 6 principles of responsible AI?
Responsible AI hinges on six key principles: fairness (avoiding bias), transparency (explainable decision-making), accountability (clear lines of responsibility), privacy (protecting sensitive data), robustness (reliable and secure systems), and human oversight (maintaining human control). These ensure AI benefits society broadly and mitigates potential harms. Striking a balance between innovation and ethical considerations is crucial in their application.
What are the 5 pillars of responsible AI?
Responsible AI rests on five key pillars: fairness, ensuring equitable outcomes for all; transparency, making AI’s decision-making processes understandable; privacy, protecting sensitive user data; robustness, building reliable and secure systems; and accountability, establishing clear lines of responsibility for AI’s actions. These pillars work together to ensure AI benefits society ethically and safely.
What is the responsible AI platform?
A responsible AI platform isn’t just a tool; it’s a system designed to minimize harm and maximize benefit from AI. It incorporates ethical considerations throughout the AI lifecycle, from data collection to deployment and monitoring, ensuring fairness, transparency, and accountability. This involves proactive measures like bias detection, explainability features, and robust security to prevent misuse. Essentially, it’s about building AI that serves humanity ethically and reliably.
How to learn responsible AI?
Responsible AI isn’t a single skill, but a mindset. It’s about understanding the ethical implications of your AI work, proactively mitigating biases, and ensuring fairness and transparency throughout the development lifecycle. This involves continuous learning through courses, research papers, and practical experience reflecting on your work’s societal impact. Finally, engaging in ethical discussions and seeking diverse perspectives is crucial.
What is responsible AI in education?
Responsible AI in education means using artificial intelligence tools ethically and effectively in teaching and learning. It prioritizes fairness, transparency, and student well-being, ensuring AI benefits all learners equally and doesn’t perpetuate existing biases. This involves careful consideration of data privacy, algorithmic accountability, and human oversight to prevent unintended consequences. Ultimately, it’s about leveraging AI’s potential while mitigating its risks for a more equitable and beneficial educational experience.
What is responsible AI in NLP?
Responsible AI in NLP means building and using natural language processing systems ethically. This involves mitigating biases present in data and algorithms, ensuring transparency in how models work, and promoting fairness and accountability in their outputs. Ultimately, it’s about designing NLP systems that benefit society without causing harm or perpetuating inequalities.
What is the responsible AI lifecycle?
The Responsible AI lifecycle isn’t just about building AI; it’s about building *ethical* AI. It’s a continuous process, from initial design considering fairness and transparency, through development and deployment, to ongoing monitoring and improvement to ensure the AI remains aligned with ethical guidelines and societal values. It’s essentially a feedback loop striving for accountability and beneficial impact.
What are the 6 principles of responsible AI?
Responsible AI boils down to six key principles: fairness (avoiding bias), transparency (explainable decisions), accountability (clear lines of responsibility), privacy (protecting sensitive data), robustness (reliable and secure systems), and human oversight (maintaining human control). These principles ensure AI benefits everyone ethically and avoids harmful consequences. Striving for these principles creates trustworthy and beneficial AI applications.
What is responsible AI in social media?
Responsible AI in social media means building and using AI systems that prioritize fairness, accountability, and transparency. This involves mitigating biases that lead to unfair content moderation or targeted advertising, ensuring user privacy, and being open about how AI algorithms make decisions. Ultimately, it’s about using AI to benefit society while minimizing harm and promoting user well-being. It’s a continuous process of improvement and ethical reflection.
What is the difference between responsible AI and generative AI?
Responsible AI focuses on building systems that are ethical, fair, and safe, addressing potential biases and harms. Generative AI, conversely, concentrates on *creating* new content like text or images. They’re not mutually exclusive; responsible AI principles *should* guide the development and deployment of generative AI to prevent misuse. Essentially, one is about ethical development, the other about creative output.